Top 5 Graph Analytics Takeaways from Gartner’s Data & Analytics Summit
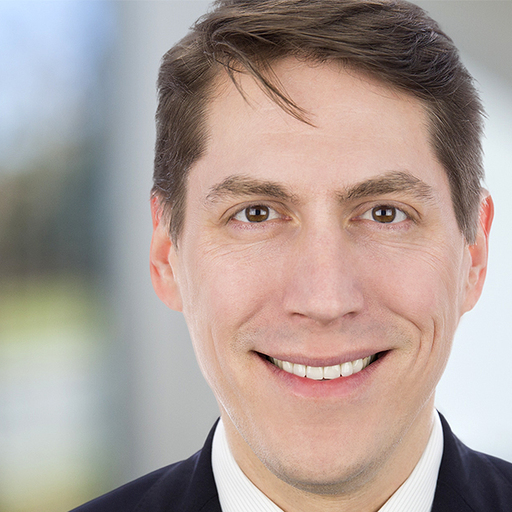
Analyst Relations
7 min read
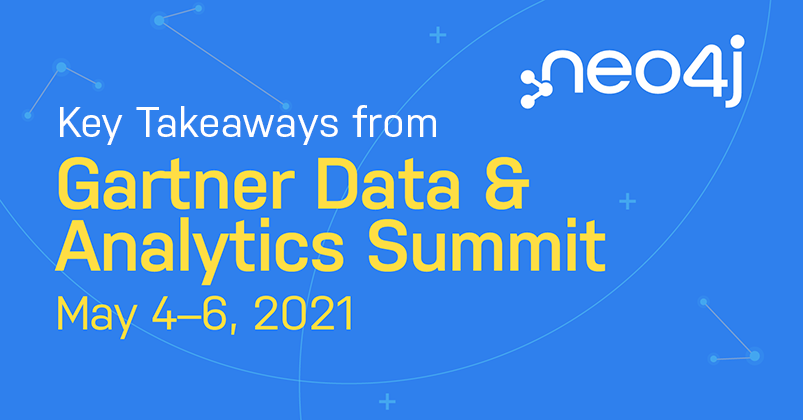
For Chief Data Officers, Advanced Analytics Leaders and Data Scientists
Graphs were everywhere at Gartner’s virtual Data & Analytics Summit, held in the Americas from May 4 – 6, 2021. From the opening keynote, through numerous vendor and analyst presentations, to the ask the expert sessions, graph was all the rage.
It’s little wonder why: Gartner predicts graph will be one of the top four enabling technologies for data and analytics along with data fabrics, generative adversarial networks, and AI autoregressive language models (source: Gareth Herschel, Research Director, Summit Opening Keynote, “Learn, Unlearn, Relearn: The Key to D&A Success,” 4 May 2021).
“The way you’ve been looking at analytics is probably only half of the way you should be looking at analytics today. Graph analytics is going to fill in some of the missing pieces in your data.”
– Jim Hare, “Connecting the Dots: Why Graph Analytics Are Key to Understanding Human and Machine Misbehavior,” 5 May 2021.
“The use of graph processing will grow at 100% annually to 2023 to enable more complex and adaptive data science.”
– Pieter den Hamer, “Graph Techniques – An Old Revolutionary Concept for Modern Data Science,” 5 May 2021
Here are my top five graph-related takeaways for data analytics leaders and scientists who couldn’t attend:
1. Graph Relates Everything
It’s a bold statement, so let’s unpack Gartner’s thinking here. One of the top trends Gartner spotted and recommends organizations seize on in their data and analytics efforts is “distributed everything.” This refers to the growing expansion of data, people, and devices happening in our lives today across the globe – especially due to the constraints of COVID-19.
Graph analytics – a sizable set of techniques that help find relationships in graphs – enable data scientists to find useful connections between all of these distributed places, things, locations, and events across diverse data. These relationships offer bottom- and top- line value to organizations because they result in improved customer understanding, service, logistics, and risk mitigation.
According to Gartner, graphs are forming the foundation of modern data analytics capabilities to such an extent that by 2021, graph technologies will be used in 80% of data and analytics innovations, up from 10% this year. (Source: Rita Sallam and Donald Feinberg. Top 10 Trends in Data and Analytics 2021. 6 May 2021).
2. Graph Creates Context Lacking in Big Data Analytics
You’ve heard that in real estate, it’s all about Location. Location. Location. In graph analytics, it’s all about Context. Context. Context.
Data leaders and scientists have long sought to better understand the connections between seemingly disparate data from fragmented assets with the goal of bringing it together to give the decision makers they serve context that is practical and actionable.
Unfortunately, siloed data and relational database approaches to make sense of that data have cut those connections and reduced context. The failure of big data analytics to drive connections and context from vast stores of data were duly noted at the summit. This has negatively impacted big data analytics efforts in many organizations.
“The real world is a giant graph. Everything is connected. When we use technologies like relational databases, it actually breaks some of the connections. We lose part of the context.”
– Jim Hare, “Connecting the Dots: Why Graph Analytics Are Key to Understanding Human and Machine Misbehavior,” 5 May 2021.
Graph is inherently superior in establishing context. One way graph technology develops deep context is through knowledge graphs. Knowledge graphs codify data, with nodes representing entities of interest and edges representing the relationships between those entities. They allow the use of connections to infer new knowledge. This enables exploration, discovery, and decision making by humans, software, or AI systems.
As Amy Hodler, Neo4j’s Director of Graph Analytics and AI Programs, noted in her summit presentation “Anticipate, Shift, Respond: The Era of Contextualized Intelligence”, Graph databases are purpose-built for relationships. “Graphs are over a thousand times faster than relational databases. Relational databases are not effective for relationships three hops out. Graph databases can do 1,000 hops out.” No disrespect meant to your favorite IPA beer, but that’s a lot of hops!
3. Graph Is Critical for AI/ML Success
Like last year’s Data and Analytics Summit, artificial intelligence (AI) and machine learning (ML) topics abounded. Augmented analytics – the use of enabling technologies like AI and ML to assist in data preparation, insight generation, and insight explanation were underscored this year as Gartner sees a clear trend in making data easier to use.
But the new story starting to emerge is how graph technology also helps analytic leaders and data scientists in these augmentation efforts using AI/ML. Not only by creating greater context (see #2 above), but by making AI more explainable.
How does graph make AI more explainable? Graph shines the light on black box neural networks and other models by helping users more easily understand feature importance. Graphs can be created that explain the relationship between features that led to the model’s conclusions in a more intuitive and accessible way.
Graph also provides metadata about the models – who touched the models, how the data was used for training, what the current version is, and more. This is especially critical for regulated industries like financial services and health care.
Neo4j’s graph platform offers native machine learning, which capitalizes on these benefits all within the database alongside integrated graph data science tools and algorithms. Data scientists can conduct the full analytical lifecycle right within Neo4j. This affords greater speed and cohesion. It helps uncover “what we don’t know we don’t know” – the patterns scientists didn’t know to look for. Graph’s ability to learn on the function of the structure rather than on specific data points helps address small and incomplete data, and helps predict missing data. Predictive models won’t age or drift as quickly because of this inherent graph benefit.
“It’s like trying to solve a Rubik’s cube puzzle by only seeing one side of it. Graph helps see all sides of it.”
– Ben Squire, Meredith, Neo4j Client
4. Graph Makes Data Science Project Management Easier and More Effective
Throughout the lifecycle of data collection, integration, preparation, exploration, feature engineering, machine learning, testing, delivery, and business process design, Graph can play a significant role in achieving efficiencies and impact.
In his presentation, “Graph Techniques – An Old Revolutionary Concept for Modern Data Science,” Pieter den Hamer notes a number of ways graph is helping advanced analytics and data science leaders achieve results:
- Accelerated Data Preparation
Most analytics practitioners will agree that munging, including integration, is one of the most time consuming activities. Graph technology can accelerate data preparation by harmonizing data formats and schema to useful models that make transformation and mapping quick and automatable. - Insightful Data Exploration through Graph Visualizations
Graph data viz allows practitioners to more intuitively and quickly find the most relevant features or input variables for prediction and classification. - Superior Feature Engineering
Graphs can enrich training data with features derived from the Graph of which data are a part. For example in centrality or influencer analysis. If another node is connected to the influencer, that fact can be used as an additional feature in the training dataset, which improves the predictive power of the ML model. - Improved Communication and Collaboration between Data Scientists and Subject Matter Experts
The use of knowledge graphs, which are a model of a knowledge domain, provide common, communicable structures, taxonomies and ontologies that yield relevant facts and contextualized answers rather than confusing and irrelevant results.
5. Graphs Will Enable the next big thing in AI – Composite AI
Gartner refers to composite AI – the combination of different AI techniques to achieve the best result – as the next “big thing” in AI. Graphs provide a common ground for the combination or composition of multiple existing and new AI techniques. Together, these composites will go beyond full data-driven machine learning. That sounds like the next quite a new-fangled ensemble model!
Conclusion
After attending Gartner’s Data and Analytics summit, it is clear that data leaders and scientists have never been more crucial for helping their organizations navigate the growth, uncertainties, and risks. Especially in the coming year.
“Most organizations don’t have a shortage of data. What they have is a shortage of understanding what is the relevant data and how to apply it to help solve the business problem… Graph allows you to create and connect all that data so that it’s available for a variety of different groups and different types of analytical needs.”
– Jim Hare, “Connecting the Dots: Why Graph Analytics Are Key to Understanding Human and Machine Misbehavior,” 5 May 2021.
Now you are armed with what more than 5,000 attendees from industries like financial services, manufacturing and government learned: graph technology has arrived and will be a key asset to organizations like yours worldwide.
And in a hopeful sign of a return to normal, Gartner has already announced next year’s Data & Analytics Summit, which is scheduled for March 14-17, 2022. In person! In Orlando, Florida! Hope to see you there!
Download the white paper The Top 10 Use Cases of Graph Database Technology for free now.