Exploring Neodash for 197M Chemical Full-Text Graph
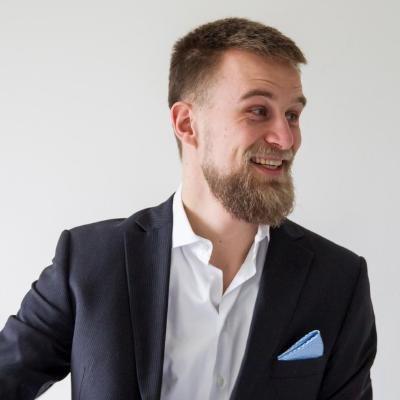
Back End Developer at CytoSMART
2 min read
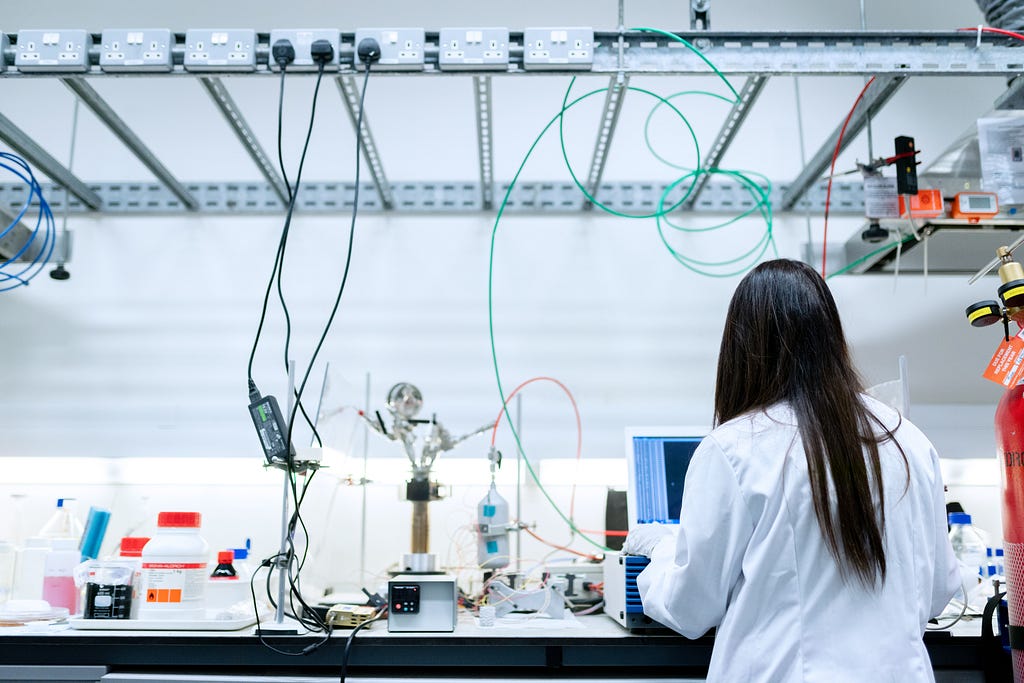
In a previous blog, I loaded 197M chemical names into a graph database. All of these are indexed with a full-text index and use the graph properties to improve the search. In order to test the user experience (not just typing queries), I built a Python backend with fastAPI and a front-end with VUE.js. This works with 2 additional languages and frameworks, but then I saw a talk by Niels de Jong at NODES 2022 about NeoDash.
NeoDash promises an easy dashboard to explore your Neo4j database, and it delivers! It is a low-code tool where I can use Cypher queries to populate blocks like line graphs, tables, maps, and more. Besides those, it also has an input field to manipulate the results as you go.
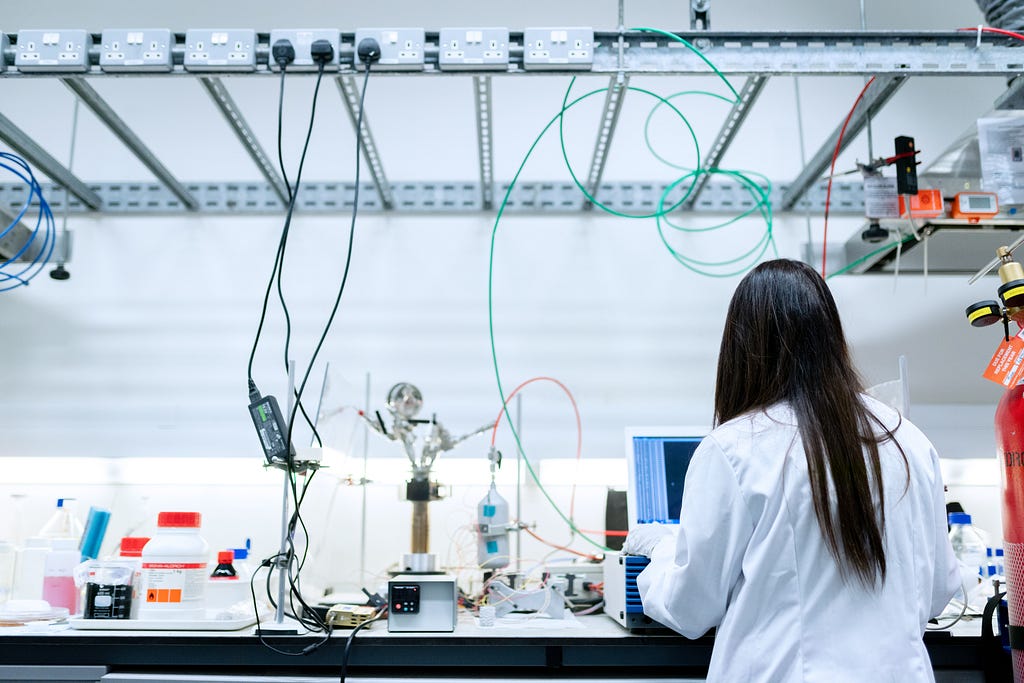
Adding NeoDash
In my current solution, I use the Python backend for some string manipulations before I use it for the Cypher query. In NeoDash, I only have Cypher, but the APOC package gives the functionality to Cypher I need.
# Python implementation
import regex as re
all_words = re.findall(r"[p{L}d]{2,}", chemical_name)
Lucende_query = "~ AND ".join(all_words) + "~"
all_words = re.findall(r”[p{L}d]{2,}”, chemical_name)
// Cypher implementation
WITH apoc.text.split($neodash_chemical_1_name, ‘[^p{L}d]’) as cleaned_input
WITH [val in cleaned_input WHERE SIZE(val) > 1] as cleaned_input
WITH (apoc.text.join(cleaned_input, '~ AND ') + "~") as lucende_query
The VUE part is easier to replace, by just creating 2 blocks, one table, and one parameter selection. This means I have an input field and a result field.
Adding the full query to the table block gives me a fuzzy full-text search on chemical names, with graph enhancements, and no additional code.
// Full chemical searching Cypher query
WITH apoc.text.split($neodash_chemical_1_name, ‘[^p{L}d]’) as cleaned_input
WITH [val in cleaned_input WHERE SIZE(val) > 1] as cleaned_input
WITH (apoc.text.join(cleaned_input, ‘~ AND ‘) + “~”) as lucende_query
CALL {
WITH lucende_query
CALL db.index.fulltext.queryNodes(“synonymsFullText”, lucende_query)
YIELD node, score
RETURN node, score limit 50
}
OPTIONAL MATCH (node)-[:IS_ATTRIBUTE_OF]->(c:Compound)
WITH DISTINCT c as c, collect({score: score, node: node})[0] as s
WITH DISTINCT s as s, collect(c.pubChemCompId) as compoundId
RETURN s.node.name as name, s.node.pubChemSynId as synonymId, compoundId, s.score as score limit 5
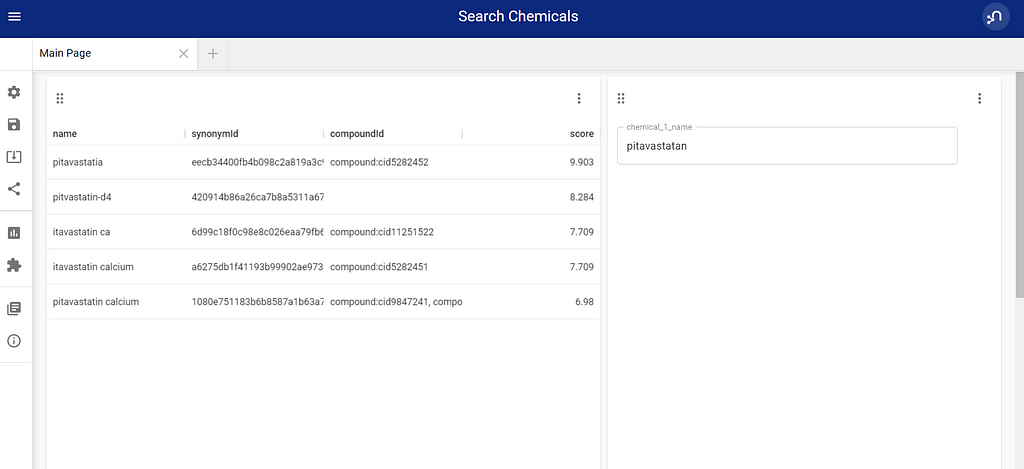
Conclusion
NeoDash works great for prototypes, and you can even host them. The setup is much easier than building your own front + backend. I will use it again for a future project.
Exploring Neodash for 197M Chemical Full-Text Graph was originally published in Neo4j Developer Blog on Medium, where people are continuing the conversation by highlighting and responding to this story.