Last time, our Junior Editor, Zaw Win Htet , shared eight questions to help you decide whether it’s time to climb onto the knowledge graph bandwagon. (I say yes for yet another reason; see below!)
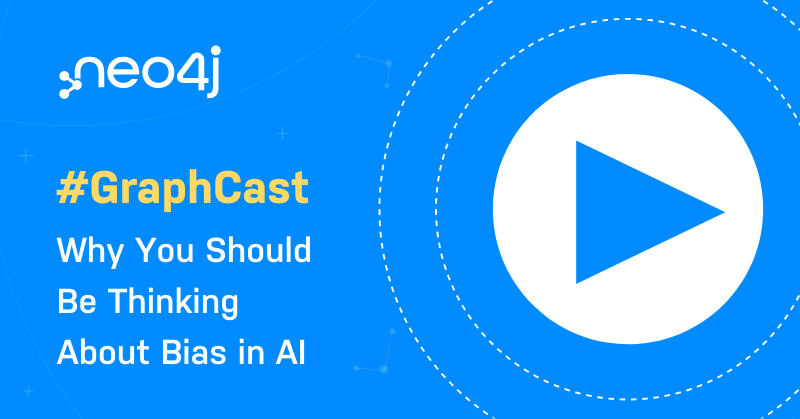
This week, I’d like to point you to this cool video on algorithmic bias in AI. It’s something we should all be thinking about because no matter what field you’re in, AI will have an impact on you in the future – if it hasn’t already!
Because machine learning depends on the data you feed it, you need to understand that data to avoid importing bias into AI. Bias can come from unrepresentative or otherwise flawed training data. Like back in 2018, when Amazon’s recruiting tool learned to discriminate against female candidates from training data that reflected an industry dominated by males.
All this brings me right back to knowledge graphs. How do you mitigate bias if you don’t even know where your data came from? Tracking data lineage in a knowledge graph is one of my favorite use cases because it also helps you be more ethical.
Catch all our videos when you subscribe to the Neo4j YouTube channel, updated weekly with tons of graph tech goods.
Subscribe Me