Presentation Summary
Welcome to the world of graphs. If this is one of your first visits and you feel like you don’t quite understand what graphs are all about, then continue reading this post.
First, we’ll get down to the basics of Neo4j, breaking down the property graph model and delving into how Neo4j differs from traditional SQL databases.
Then, we’ll introduce the one slide – the secret sauce of Neo4j – that you must understand. Along with that, we’ll explore modeling by looking at examples on modeling flight data, building a news feed and constructing a recommendation engine.
Full Presentation: The Secret Sauce of Neo4j
My name is Max De Marzi and I’m a Neo4j Field Engineer. I blog about Neo4j and all kinds of crazy things you can do with it. I also go to different customer sites, where I help them solve their problems and build a graph solution.
This post is mostly about three corners of my experience with Neo4j, but what matters from this post is that you click out thinking about graphs. You have to drink the Kool-Aid, become a graph person, make it a part of your lifestyle and forget about all the other bad databases that exist out there. That’s my goal.
The Property Graph Model
Let’s first talk about a property graph model, which is where things start. In graph databases, we don’t have tables, columns, rows or foreign keys. Instead, all we have are objects floating around in space, connected to each other by relationships that are directed and have types.
For example, we see here that Mary drives the car; the car doesn’t drive Mary. Also, Mary loves James and he loves her, but that doesn’t necessarily have to be the case.
Moreover, the properties can be different. Here, we have two people or user nodes, but James has a Twitter property while Mary doesn’t. That’s also perfectly valid.
The other thing to notice is that the relationships also have properties, unlike the triplestore or RDF model, where relationships don’t have any properties. We also have labels; nodes can have one or more labels that identify what kind of node it is. These labels are optional, but nowadays everyone generally has at least one label on a node.
What You (Probably) Already Know
If you come from a SQL background, you probably already know a lot about tables.
Say I have a customer with an address. With SQL, I’d have a customer table and an address table, and I’d connect the two together with the foreign key. Everyone’s happy.
But that’s not all that happens in the real world. For example, if a customer can change an address, I’d now need to have a pointer going the other way from the address table to the customer table. But, one address can have multiple customers and one customer can have multiple addresses, and it gets a little messy, so we utilize a join table. This gives us a customer address table that points both ways. It has no practical value except providing the foreign keys where it points to.
In this way, if I wanted a query on how this data is related, I’d have to perform some kind of join. And that’s the way we’ve been doing it for more than 30 years in SQL. The problem here is that every time you want to know how things are connected, I’d have to execute that join. What that really means is that I’d need to find those foreign keys, see where they’re connected and follow them through.
How do you find the key in a relational database? Well, I’d have to use an index. And if my database people did their job properly, everything should be nicely indexed, meaning that I’d have a B-Tree in the database. A B-Tree’s is a log n data structure, which means as my data grows by 10, speed only slows down by two. This means we can get pretty big before encountering problems.
But, the problem is that we already got to that large size. With this big data, we’re searching for more keys, meaning we get slower and slower performance. The more joins and look-ups for keys we have to do, the worse things get. We start screaming at our database to go faster – and that’s usually when we transition to NoSQL.
Same Data, Different Layout
The folks at Neo4j took the same data you had and gave it a slightly different layout. Let’s look at some conference data:
If we’re only focused on which conference Max, for example, attended, we realized we didn’t don’t care who the next person is or what other conferences exist in the tables. All I care about is the relationships between everything. Its data is still there, we just don’t need tables to handle this. Instead, we use nodes and relationships.
Relational Databases
Even though they’re called relational databases, they really can’t handle relationships. As you add more and more relationships to your relational database, it gets more and more complex, and it starts looking crazy. Sometimes, you’ll walk into a DBA or developer room, see ginormous ERD diagrams that take up the whole wall and you’re supposed to understand what’s going on with that. It’s very hard to do. Again, as your data grows with the number of joins and you try to execute a table join that’s 15, 20 or 30 levels deep – forget it. It’s not going to be a good time.
SQL was built so many years ago around Set Theory, not graph theory. So if the data feels, smells and tastes like a graph, using SQL on it is the wrong thing to do. (Don’t get me wrong: SQL is great if you have nothing but tabular or set data.)
Moreover, SQL isn’t very flexible, which means that you have to know your schema before adding any data.
NoSQL Databases
To fight these problems, a bunch of vendors got together under the moniker of NoSQL, which includes the following major categories:
Starting from the bottom, we have key-value stores, which helped fix the join problem.
Next, we have the column family, which says: “Listen, we’re going to pre-join everything for you, duplicate the crap out of your data and you’re going to buy 100 servers from us. So we’ll make lots of money.” In many cases, you’ll end up with thousands of servers at Apple running Cassandra and stuff like that.
Document databases say: “That’s a horrible idea. We have a better idea. We’re going to take everything we know about an object and squish it all together.” This is fine, unless you want to know how these documents are talking or connected to each other.
Last, you have the graph database saying: “Let’s take relationships and make them first-class citizens. We’re going to store them on one disk and keep them up in memory. They’ll be physical, real things in our data.
Now, the bottom three categories – document stores, column stores and key-value stores – typically aren’t ACID; they’re non-transactional, though some have started to announce that they’re becoming proper ACID databases. Additionally, most of these can’t handle relationships very well.
What’s Our Secret Sauce?
Our secret sauce is here. If you have no idea what graphs are or what Neo4j is about, this is the thing to remember, though it’s not a perfect, physical description of how it works, but rather a slight extraction.
Basically, what you have is two giant arrays: one array of nodes and one array of relationships. Every record in the array is fixed in size, so you’re actually looking at one single node and one single relationship of the tree.
Furthermore, every node knows what types of relationships are connected to it. Let’s say we’re looking at a social network and you have to be in Node One. The node knows it has friends relationships that happen to be of Type One. If we want to get all their friends, we can go to the Type One relationship and ignore the Type Two and Type Three relationships.
Then, every relationship type has two lists – incoming and outgoing – that contain relationship IDs, which are basically relationship pointers. What’s nice about this is that every type list is stored by node, so whether you have a thousand or a billion people in your graph, getting through 150 friends costs exactly the same. You essentially jump to the node you care about, traverse the 150 relationships and you’re done. All it cares about is what you’re looking at.
However, we pay a price: the joins are done on creation. What happens every time we create a relationship? We need to go to the first node and add an item to its list in the outgoing direction. Then we go to the node that’s receiving the relationship – to the list of incoming – and we add an entry there. After, we go to the actual relationship object and save its direction, and also add some properties if necessary. In this way, it costs us three, rather than one, to add a relationship to Neo4j. In contrast, in a relational database, you go to the join table, get an entry with two numbers in it and you’re done.
But an upside is that we get the joins for free. Everything is done by an array, so there’s no hatching, look-ups or indexing. Everything is done directly by O1 access, by looking at the next array. These relationships just store an ID, which points to the record.
If you get this, you’ll understand what Neo4j is about, regardless of size. It’s just a matter of traversing relationships using arrays. There’s no magic to it – you can build this in a weekend.
Real-Time Query Performance
What this provides are very fast queries for some use cases, but not for everything. Like many databases, Neo4j is good at some things and terrible at others.
Let’s go through some bad queries. Imagine you have the data of every actor in Hollywood with everyone’s height property. Say you wanted to figure out the average height of all of the actors. That’s a terrible query for Neo4j, because we’d have to jump around random nodes looking for those actors. The first node could be an actor, the second node could be a movie, the third movie could be a commercial, the fourth node could be a play – who knows? Obviously, you might have labels that tell you where the actors are, but you still need to jump around quite a bit.
Next, you have to find the height property. Some actors might have the height property as their first property and some might have their height property as their last, because nodes can have any number of properties, in any order whatsoever. All of this is going to cost us a lot more than a relational database, which can just go to the actor table and do a scan, or a column sort that can go into height, go across and figure out the average. This is a better query for Cassandra than anything else.
However, if you wanted to know how these actors were connected to each other by the movies that they were in, then that’s a perfect query for us. Same data, but a different use case and query. If you care about performance, you want to take all of this into account.
Reimagine Your Data as a Graph
It all works as long as you can reimagine your data as a graph. You’ll get better performance if you’re not only looking at sets of data, but also its relationships.
Cypher was built for graphs, so it’s really easy to explain what you’re trying to do with a language like Cypher. However, if you can’t do it with Cypher, you can do it with Java, Gremlin or other languages that work with Neo4j.
Moreover, Neo4j is flexible and consistent – you can add data, nodes and properties on the fly. It’ll figure out whether something’s a string, a number or a user. Neo4j will just store it as whatever you give it; it’s schema-optional in a way. This works really well for proof of concepts or when you have a greenfield idea and not sure what you’re going to build.
But, it’s also consistent because we have real transactions. You can start a transaction, make a thousand changes to the graph all over the place and at the end decide to commit or rollback all of those changes. In this way, it’s very similar to your relational database.
Modeling
This is where things get weird. If you know the third normal form or traditional database modeling, you’ll need to unlearn a few things.
Of course, there’s the normal way of doing it, and we always tell people this first lie: that graphs are completely whiteboard friendly. And they are – if you want to go slow. But if you want to go fast, you need to get a little more creative.
Some models are easy, like this one:
If you wanted to know who played James Bond, this is a terrible model for that query, because there’s no quick way to get to it. You’d have to rip out the actor or character, turn that into a separate node, find the James Bond character and figure out who played that character and what additional movies they were in. In this way, the models and the queries have to align. It’s not like the normal form where this is the right way to model things, and we’ll just figure out where the queries are later; you have to build it from both sides. You need to build it from the data you have, the queries you’re asking and match the two together to get the right answer.
Flight Data
I’ll quickly go through another example of how to model flight data and airlines. You could model it like this:
We have an airport – with a name and a code – as well as a flight to another airport, who has its own name and code, and we’ll stick everything about the flight into the relationship.
Let’s say I want to build a flight search engine, and want to find flights from New York to Chicago tomorrow. This is a horrible model for that because I’d have to look at the JFK airport, find all the flights for the whole year, look for the ones that are tomorrow and see which ones are specifically going to Chicago. That would take forever.
Another thing we can do is make flight a node. That way, the airport has a flight, the flight has a destination and we’ll stick with the properties in there:
Okay. Things got better but didn’t get any faster. In fact, it’s actually slower, because now we have to jump through two nodes.
So, we have to get creative. Well, people are gonna be searching by date, so we can take the airport model and say the airport has days. Every day has flights, so instead of looking at 300,000 flights in a whole year, I can just look at the 1,000 flights in a single day:
This made my query better because now I have to look at less of the graph. But, we can still do better than this. Instead of having the
HAS_DAY
relationship, we can actually put the date as the relationship type. Now we can go from the airport through this dated relationship directly to the airport day. We can even skip looking at the 365 days and go directly, but that doesn’t really buy me much – it only buys me 365 relationships.
I can make this even faster by getting rid of the airport altogether and labeling the node as
code-date
, so for every airport, it’ll have many of these airport days: By now, I’ve already shown you four different ways to model the same data and they’re getting weirder and weirder. Let’s keep going and get a little more creative. Say an
AirportDay
has 1,000 flights, but only 100 destinations – 20 to Chicago. What we can do is create a Destination
node and indicate that this AirportDay
has a destination to Chicago. This way, the Destination
node would only have 20 flights. Now I need to look at less of the graph to get the answer, and my query will go faster by creating this
Destination
node out of thin air. But I can get even more creative by taking the
AirportDay
and creating a relationship type that contains the code of the airport. Now this AirportDay
will look at the flights going to Chicago, go out that direction, get to the flight and continue on from there. By using this model, I can skip looking at any flights that go to any destinations that aren’t Chicago. We’re actually promoting a property of the relationship forward so we can skip looking at the part of the graph we don’t really care about.
Why are we doing this? Because every node knows what types of relationships are connected to it, so if we tell it to “go out to the Chicago destination flight relationship,” we can skip everything else. Now, we’re looking at 20 flights, which is going to be incredibly faster than looking at 1,000 flights in a day or 300,000 flights in a year.
The thing to understand here is that there’s no right way to model data in a graph, though there are plenty of wrong ways. You have to get creative and find proper solutions by changing the data a little bit.
If you think this is all insane, take a look at what the folks from Marvel Entertainment have done. In GraphConnect 2013, Peter Olson came along and essentially said: “We have a very complex data model – this is the fantasy world where anything can happen. People go back into the past, into the future and have alternate timelines. How do you model that?” They figured out how to do it in Neo4j using hyperedges. Check it out – it’ll teach you everything you need to know about modeling complex domains. If they can model the fantasy world, you can model the real world.
More Modeling: Building a News Feed
Let’s talk a little more about modeling, just to drive you guys a little crazy. Say you want to clone Twitter. How would you do something like this in a graph in Neo4j?
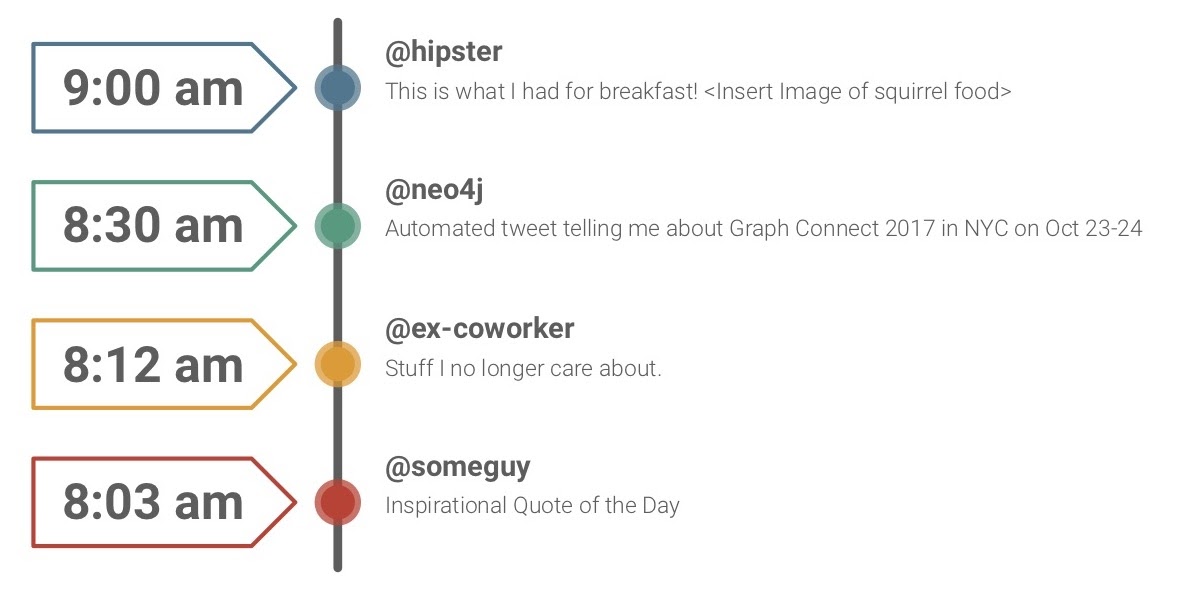